Models can convey biases and false information to users
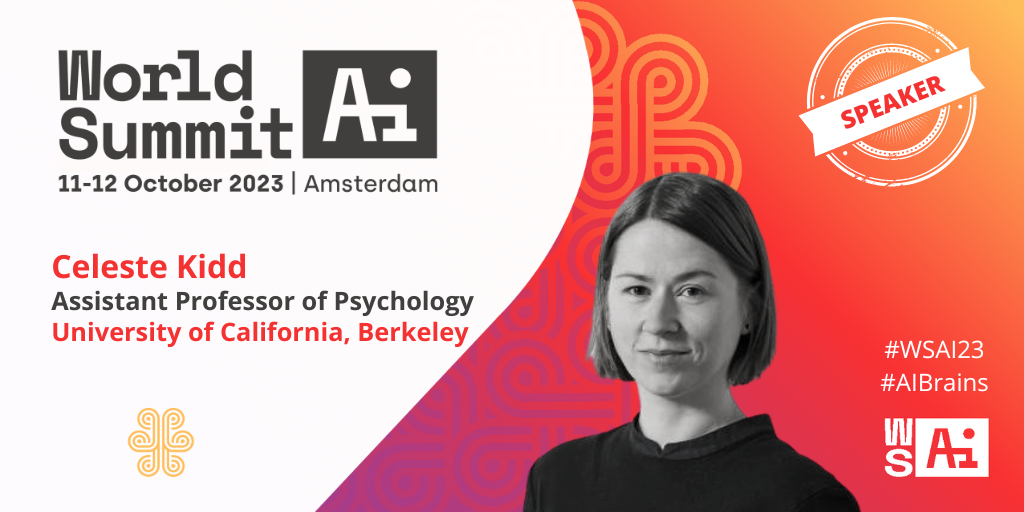
By Celeste Kidd(1) and Abeba Birhane (2-3)
Individual humans form their beliefs by sampling a small subset of the available data in the world. Once those beliefs are formed with high certainty, they can become stubborn to revise. Fabrication and bias in generative artificial intelligence (AI) models are established phenomena that can occur as part of regular system use, in the absence of any malevolent forces seeking to push bias or disinformation. However, transmission of false information and bias from these models to people has been prominently absent from the discourse.
Overhyped, unrealistic, and exaggerated capabilities permeate how generative AI models are presented, which contributes to the popular misconception that these models exceed human-level reasoning and exacerbates the risk of transmission of false information and negative stereotypes to people. Generative AI models—including OpenAI’s GPT variants, Google’s Bard, OpenAI’s DALL·E, Stable Diffusion, and Midjourney—have captured the minds of the public and inspired widespread adoption. Yet, these models contain known racial, gender, and class stereotypes and biases from their training data and other structural factors, which downstream into model outputs (1–3).
Marginalized groups are the most negatively affected by these biases. Further, these models regularly fabricate information (4). Some model developers have acknowledged these problems but suggested that people must use the systems to reveal trends in problematic outputs to remedy them. This ignores that distortions to human beliefs caused by generative AI models cannot be easily corrected after problems are discovered.
Further, the reactive nature of this approach does not acknowledge a key problem of current generative AI systems, the inability of their architecture to distinguish fact from fiction (4). Three core tenets of human psychology can help build a bridge of understanding about what is at stake when discussing regulation and policy options. These ideas in psychology can connect to machine learning but also those in political science, education, communication, and the other fields that are considering the impact of bias and misinformation on population-level beliefs. People form stronger, longer-lasting beliefs when they receive information from agents that they judge to be confident and knowledgeable, starting in early childhood.
For example, children learned better when they learned from an agent who asserted their knowledgeability in the domain as compared with one who did not (5). That very young children track agents’ knowledgeability and use it to inform their beliefs and exploratory behavior supports the theory that this ability reflects an evolved capacity central to our species’ knowledge development. Although humans sometimes communicate false or biased information, the rate of human errors would be an inappropriate baseline for judging AI because of fundamental differences in the types of exchanges between generative AI and people versus people and people. For example, people regularly communicate uncertainty through phrases such as “I think,” response delays, corrections, and speech disfluencies. By contrast, generative models unilaterally generate confident, fluent responses with no uncertainty representations nor the ability to communicate their absence. This lack of uncertainty signals in generative models could cause greater distortion compared with human inputs.
Further, people assign agency and intentionality readily. In a classic study, people read intentionality into the movements of simple animated geometric shapes (6). Likewise, people commonly read intentionality—and humanlike intelligence or emergent sentience—into generative models even though these attributes are unsubstantiated (7). This readiness to perceive generative models as knowledgeable, intentional agents implies a readiness to adopt the information that they provide more rapidly and with greater certainty. This tendency may be further strengthened because models support multimodal interactions that allow users to ask models to perform actions like “see,” “draw,” and “speak” that are associated with intentional agents. The potential influence of models’ problematic outputs on human beliefs thus exceeds what is typically observed for the influence of other forms of algorithmic content suggestion such as search. These issues are exacerbated by financial and liability interests incentivizing companies to anthropomorphize generative models as intelligent, sentient, empathetic, or even childlike.
The number of exposures to fabricated information predicts how deeply ingrained the belief in that information becomes. Greater repetition predicted greater strength in a person’s belief in a false statement—even when the statement contradicts a person’s prior knowledge (8). Trends that increase people’s exposures to fabrications consequently increase the strength of people’s beliefs in false information. The trend of integrating generative AI models into existing technologies—e.g., search engines and smartphones—will almost certainly mean greater exposure to the models’ fabrications and biases.
Similarly, repeated exposure to biases in algorithmic systems transmits the biases to human users over time. For example, when a risk-assessment system, such as used by court judges to determine how a defendant
should be sentenced (9), assigns Black individuals higher risk scores than white individuals with the exact same criminal histories, human judges learn these statistical regularities and may “change their sentencing practices in order to match the predictions of the algorithms” through a process likened to anchoring [(10), p. 287].
This mechanism of statistical learning could lead a judge to believe Black individuals to be more likely to reoffend—even if use of the system is stopped by regulations like those adopted in California. Generative AI models have the potential to further amplify the repeated exposure issues for both fabrications and bias because of their expected influence on contents of the World Wide Web—a primary source of training data
for the models. For example, the rapid rise and accessibility of generative models, such as Stable Diffusion, have generated millions of outputs each day (11). This output in turn becomes part of the training data for the next generation of models—thus amplifying the impact of the systemic distortions and biases into the future in a continuous feedback loop.
The more rapidly such systems are used and adopted, and the more they are built into the backend of systems used across all sectors, the more influence the systems have over human beliefs. For example, marketing content can now be generated by generative AI models, then targeted at users using psychometric methods, then fine-tuned, looped, and fed back to users in an automatic system designed to induce engagement behaviors, irrespective of and incapable of considering how its content might distort human beliefs in general or the inclusion of either fabrications or stereotyped biases in this material.
Users of conversational generative AI models request information in particular moments—when they are uncertain and thus most open to learning something new. Once a person has received an answer, their uncertainty drops, their curiosity is diminished, and they don’t consider or weigh subsequent evidence in the same way as when they were in the early stages of making up their minds (12). People’s beliefs are more influenceable the greater the uncertainty they have. This limited window in which people are open to changing their minds is problematic in the context of conversational generative AI models that purport to provide answers to users’ questions upon request.
This aspect of human curiosity has longstanding implications for how these systems affect human beliefs. It means that information transmitted from a large-scale language model to an uncertain person will be difficult to update after the fact—because the information provided by the model will resolve the person’s uncertainty even if it is incorrect (13). The problems also affect the use of systems that generate images from users’ text prompts because the act of asking a model to translate text into visual imagery can be driven by curiosity that resolves once the user sees the visual output. Negative sterotyped biases in such visual outputs run similar risks of taking root in stubborn ways. Once a faulty belief is fixed within a person—and especially if the same fabrication or bias is passed and then becomes fixed in many people who use the same system—it can pass among people in the population in perpetuity (14).
Thus, transmitted biases or fabricated information are not easily correctable after the fact either within individuals or at the population level (15). This aspect of human psychology interacts with how humans treat agentive entities and, in particular, their tendency to be more greatly swayed by agents that they perceive as confident and knowledgeable (6). The amount of information required to reach that threshold certainty will be less in the context of it being delivered by a seemingly confident and knowledgeable agent—especially if it is presented in morehumanlike ways, as in the context of a conversation.
Thus, developers’ claims surrounding their generative AI system can affect how much faulty outputs distort human beliefs. The nascent stage of this technology offers a transient opportunity to conduct interdisciplinary studies that measure the impact of generative models on human beliefs and biases. This opportunity rapidly diminishes once these systems are more widely adopted and more deeply embedded into other everyday technologies. Research on how generative AI models affect children’s beliefs is an especially high priority.
Children are more vulnerable to belief distortion because of their increased tendencies to anthropomorphize technology and their more nascent, influenceable knowledge states. Independent audits must include not only assessments of fabrication and bias but also measurements of how knowledgeable users rate systems to be and how much they trust the outputs. These data could be used to estimate both the rate of problematic model outputs to users and how severely these outputs influence human beliefs in advance of actual transmission. The fields of psychology and machine learning could unite to turn their attention, collaborative capacities, and resources to doing this work.
Studies and subsequent interventions would be most effectively focused on impacts on marginalized populations who are disproportionately affected by both fabrications and negative stereotypes in model outputs. Resources are needed for the education of the public, policy-makers, and interdisciplinary scientists to give realistically informed views of how generative AI models work and to correct existing misinformation and hype surrounding these new technologies. Collaborative action requires teaching everyone how to discriminate actual from imagined capabilities of new technologies to focus on tackling real,
concrete challenges together.
REFERENCES AND NOTES
1. A. Birhane, Nature610, 451 (2022).
2. A. Birhane, Patterns2, 100205 (2021).
3. E. M. Bender, T. Gebru, A. McMillan-Major, S. Shmitchell,
in Proceedings of the 2021 ACM Conference on Fairness,
Accountability, and Transparency (Association for
Computing Machinery, 2021), pp. 610–623.
4. R. Azamfirei, S. R. Kudchadkar, J. Fackler, Crit. Care27, 120
(2023).
5. M. A. Sabbagh, D. A. Baldwin, Child Dev. 72, 1054 (2001).
6. F. Heider, M. Simmel, Am. J. Psychol. 57, 243 (1944).
7. A. Birhane, J. van Dijk, in Proceedings of the AAAI/ACM
Conference on AI, Ethics, and Society (Association for the
Advancement of Artificial Intelligence, 2020), pp. 207–213.
8. L. K. Fazio, R. M. Pillai, D. Patel, J. Exp. Psychol.151, 2604
(2022).
9. B. Green, Y. Chen, in Proceedings of the 2019 ACM
Conference on Fairness, Accountability, and Transparency
(Association for Computing Machinery, 2019), pp. 90–99.
10. A. Christin, in The Decisionist Imagination: Sovereignty,
Social Science and Democracy in the 20th Century, D.
Besser, N. Guilhot, Eds. (Berghahn Books, 2018), pp.
272–294.
11. F. Bianchi et al., https://arxiv.org/abs/2211.03759 (2022).
12. L. Martí, F. Mollica, S. Piantadosi, C. Kidd, Open Mind2, 47
(2018).
13. S. Wade, C. Kidd, Psychon. Bull. Rev. 26, 1377 (2019).
14. C. Kidd, B. Y. Hayden, Neuron88, 449 (2015).
15. B. Thompson, T. L. Griffiths, Proc. R. Soc. B288, 20202752
(2021).
ACKNOWLEDGMENTS
The authors thank Ş. Wong, D. Raji, C. O’Neil, A. Smart, T. Ryan, B. Thompson, A. Eason, S. T. Piantadosi, and two reviewers for feedback. C.K. is supported by the Walton Family Foundation, the Hellman Fellows Fund, DARPA Machine Common Sense TA1 (BAA no. HR001119S0005), and the John Templeton Foundation (Character Virtue Development, ID 61475). A.B. is supported by Mozilla Senior Fellows, Trustworthy AI.
10.1126/science.adi0248
Global AI events calendar
Montréal, Canada