Predictive Maintenance Made Possible by AI Will Go Mainstream: Here’s What Business Leaders Need to Know. We share lessons learned about setting up predictive maintenance solutions in enterprise.
Tailor services to your needs. Predict the future. Automate away tedious tasks so you can focus on the important stuff.
These are the most common hyped abilities of machine learning on the market today. Every day, however, we see how much-hyped technologies increasingly become part and parcel of products we adore. Tailoring streamed video suggestions to your tastes via a recommendation system; algorithms that predict future harvest yields based on data of growing conditions; chatbots that make navigating the bureaucracy of city hall less of a befuddling affair. These common examples show the growing value businesses can accrue from AI in the here and now. Get ready to add another item to the list: predictive maintenance. Made possible by AI, the technology recently transitioned from theoretical to commercial applications [1], whereby its initial uptake demonstrates why it merits being on the radar of business leaders.
Predictive — sometimes described as preemptive — maintenance is already employed in various forms by a handful of intrepid companies and new entrepreneurial ventures. Consider the Montreal start-up Canscan that employs computer vision technology to identify when individual shipping containers have too much wear-and-tear by identifying areas with excessive corrosion or punctures. Also check out what’s happening at the major British online grocer, Ocado. Their use of wheeled robots to move food orders smoothly through expansive distribution warehouses recently caught the eye of The Economist magazine. They report that by using basic data in the form of energy consumption metrics and torque readings from the wheel motors, the company trained machine-learning models to predict when an individual robot was at risk of a mechanical failure before it happened. These two tangible examples show the potential for these emerging technologies to keep operations running smoothly. Expect this high-tech tactic to soon expand into more complex applications.
How best to implement a top-notch predictive maintenance system within an industrial process or large enterprise remains a work in progress — being a process with which we have first-hand experience. Here we share a handful of key insights we continue to learn as we at Zetane implement the technology for fleets of heavy-duty vehicles. Our goal is to highlight the important components required to build a robust predictive maintenance solution and strategies for avoiding common challenges with its implementation. We begin with an overview of the potential predictive maintenance has for several prominent industries.
This high-tech method to better manage maintenance operations appears to be increasingly popular among those with deep pockets. Multiple key market indicators demonstrate that the commercial potential of predictive maintenance services is strong on a global level. From 2019 to 2024, the global market for predictive maintenance is estimated to grow at a cumulative annual growth rate of 28.8%-30.2% to reach $10.7-$23 billion UDS, where its application in defense and transportation will be particularly significant [2,3]. Current estimates value the global predictive maintenance market at over $2.8 billion USD, where North American companies lead in demand for the technology [4].
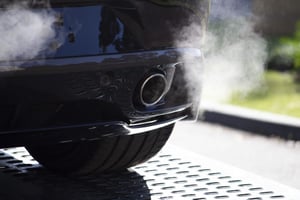
The expected advantages of predictive maintenance are significant. Past studies from the United States Department of Energy (USDE) [6] estimate that in comparison with current preventative maintenance solutions, new predictive maintenance solutions can reduce associated costs by 8%-12%. On its own, predictive maintenance solutions can achieve 30%-40% cost reductions by reducing maintenance costs, avoiding breakdowns, avoiding ‘downtime’ and project delays, and increasing productivity. The studies by the USDE estimate predictive maintenance solutions can accrue a return on investment (ROI) up to 10 times the investment in the technology, which is astounding.
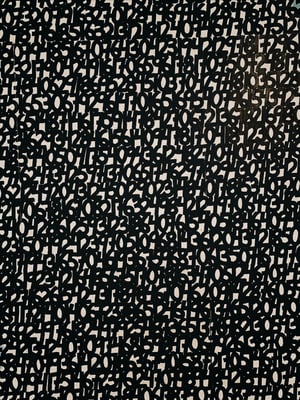
Additional benefits pertain to the ability to significantly upgrade the management of maintenance operations. The data crunching and assessments made by predictive maintenance algorithms are often basic metrics that are easy to relay to a command-and-control centre. Displays of the information for both individual vehicles and entire fleets is now possible, enabling a company to monitor the maintenance needs of their machinery in real-time. Imagine how this can increase efficiencies compared to current maintenance operations. For one, the technology makes it possible for fewer employees to effectively oversee and manage maintenance operations for a corporation, even for fleets of vehicles in multiple locations.
Another essential component of predictive maintenance solutions includes the aforementioned computer vision technology (a.k.a. “machine vision”). AI-powered abilities to “see the world” provides means to automate some tasks related to the routine inspection of vehicles that previously required a highly-trained professional to complete. The increasingly common technology can analyse videos and images of vehicles and alert officials of suspected problems. Common features include abilities to identify unlatched hinges for doors, punctures, corrosion, misaligned tires, distorted and damaged parts, and more.
Together, the three components of:
1) predictive algorithms made from historical data,
2) automated inspections by computer vision and
3) a control centre
Predictive maintenance is far from being all golden and rosy. Let’s not kid ourselves about the novelty of the technology; meaning, our abilities to implement and reap the benefits of predictive maintenance solutions in diverse sectors has yet to be seen. Being an AI-based innovation, we cannot ignore the many critiques that surround efforts to introduce AI in business [7,8,9]. This article began by noting AI is all-too-often hyped and provides no guarantee of bringing to light unseen efficiencies and bountiful profits [10]. Just two years ago, the respected technology assessments provided by Gartner assessed many AI innovations as being on the verge of tumbling into their infamous “trough of disillusionment” [11]. Indeed, applying AI to the real-world application of predictive maintenance is cutting-edge, and thus previous examples that demonstrate a significant return on investment in any given industry vertical remain limited (the few known ROI assessments we could find are for a handful of industrial verticals indicated in: [12]). The point here is not to fear innovation; rather, business leaders need to have reasonable expectations. Yes, the potential for predictive maintenance is huge, but likely not equivalent for all adopters of the technology. And it undoubtedly comes with some risk — but that can be said of just about any new technology.
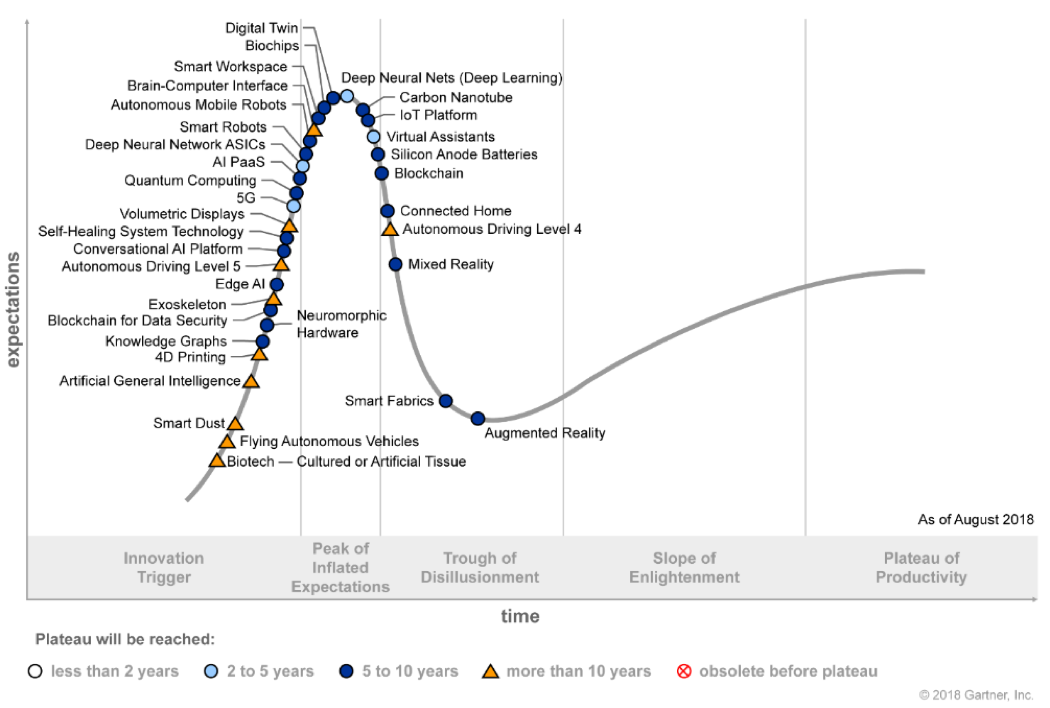
It doesn’t need to be this way and you should ask to see “human-understandable” versions of the technology before signing on the dotted line. Request from your AI service provider for more thought out descriptions of the final product. Ask if it can be represented in scenarios or simulations, as a minimal viable product (MVP), or as a prototype that your tech team can review. Note that these more intuitive representations of AI technology may slightly increase costs and the time to complete the project, but we argue this reasonable expense is well worth the investment.
Any AI project is only as good as the data used to build it, which raises a second key risk that should be on your radar. Senior executives at IBM state that data-related challenges are a primary reason why their corporate clients seeking AI services abandon or shy away from AI projects [13]. Some businesses already have banks of neatly organized data, such as the aforementioned case with Odesa’s wheeled robots. Many companies are less fortunate. The rise of data-collecting sensors and equipment from the internet of things (IoT), however, continues to make diverse data available. How has your business embraced IoT, if at all? Historical data of past maintenance schedules abound within industries where safety and good operating practices are heavily regulated, such as in aviation and businesses operating passenger transport vehicles. If your industry falls outside this category, you may need to produce synthetic data using simulations, and that comes with added costs. Once again, we propose that a good means to estimate whether your organization has sufficient quality data for predictive maintenance solutions is to build an MVP or a prototype with the data you have on hand. Use this prototyping period to also assess whether your AI service provider has a big enough team to process your data within reasonable time limits, and align your expectations from those assessments accordingly.
Skimming over the details of the command-and-control centre for predictive maintenance is unwise. Indeed, the main purpose of the command centre is to provide a practical means to better manage maintenance operations; also of import is knowing how well the command centre can integrate into your pre-existing workflows and provides means to introduce novel AI products into your business smoothly. Overall, the command centre should be a bridge between a working AI model and a useful operating system in an enterprise environment. To put this into perspective, consider an example from finance. The fraud-detecting Falcon algorithm provided a major improvement in flagging suspicious transactions. The technology languished until its developer provided banks with a management system that could relay the outputs from the algorithm to bank employees who would then block or allow specific transactions.
How confident would you feel when deploying a technology if you had little understanding of its inner workings? Hesitations on this front are reasonable, as well as common within the AI sector. Given the complexity of AI technology, understanding what goes on inside AI models proves challenging, being what the industry describes as the “black-box algorithm” problem. As a final noteworthy risk, business leaders need to set limits on just how nebulous their predictive maintenance solutions can be based on reasonable industry standards. This threshold is low, for example, if machinery and fleets of vehicles are operating within “mission-critical contexts” such that a minor blip in an AI prediction can balloon into a major thorn in one’s side. Greater confidence can come partly from assessing the inner workings of the AI technology using explainable AI tools. Tools to conduct such assessments are increasingly common, often grouped under the heading of xAI Python Libraries, which can be particularly insightful in computer vision projects. Working with your AI service provider, we recommend defining methods using these tools to peer inside AI algorithms so various stakeholders can conduct safety audits and assess the fundamental workings of the predictive maintenance solution. From our experience, analysing feature maps of artificial neural networks within algorithms and employing common tools in xAI, such as LIME and Grad-CAM, are a great way to start shining light into the black box.
- A systematic literature review of machine learning methods applied to predictive maintenance (2019) Computers & Industrial Engineering; Volume 137, p.106024
- Predictive Maintenance Market by Component (Solutions and Services), Deployment Mode, Organization Size, Vertical (Government and Defense, Manufacturing, Energy and Utilities, Transportation and Logistics), and Region — Global Forecast to 2024 (2019) Markets and Markets
- Predictive Maintenance Market to Garner $23,014.7 Million at a CAGR of 30.20% by 2026 (2019) Press release from Big Market Research
- Predictive Maintenance Market by Component (Software and Service), Deployment Type (Cloud and On-premise), Technique (Vibration Monitoring, Electrical Testing, Oil Analysis, Ultrasonic Leak Detectors, Shock Pulse, Infrared, and Others), Stakeholder (MRO, OEM/ODM, and Technology Integrators), and Industry Vertical (Manufacturing, Energy & Utilities, Aerospace & Defense, Transportation & Logistics, Government, and Others): Global Opportunity Analysis and Industry Forecast, 2019–2026 (2019) Allied Market Research
- A Critical Review and Analysis of Construction equipment emission factors (2017) Procedia Engineering; Volume 196, p. 351–358
- Chapter 5 of: O&M Best Practices Guide, v3.0
- The cost of training machines is becoming a problem. The Economist; Technology Quarterly
- Businesses are finding AI hard to adopt. The Economist; Technology Quarterly
- For AI, data are harder to come by than you think. The Economist; Technology Quarterly
- An understanding of AI’s limitations is starting to sink in. The Economist; Technology Quarterly
- Gartner Identifies Five Emerging Technology Trends That Will Blur the Lines Between Human and Machine. Press release from gartner, 2018
- Predictive Maintenance: Increasing Your Equipment’s ROI. Gutenberg Technology
- Data Challenges Are Halting AI Projects, IBM Executive Says (2019) Wall Street Journal
- 2020 AI Predictions: Get on board with boring AI. PwC
Here is your Global AI Events Calendar where you can meet your fellow 54,000 InspiredMinds community members of business leaders, heads of government, policy makers, startups, investors, academics and media.
WORLD SUMMIT AI WEBINARS
INTELLIGENT HEALTH
9-10 September 2020
Online
INTELLIGENT HEALTH AI WEBINARS
INSPIRED AI SERIES
Sept – Nov 2020
Online
inspired-minds.co.uk/inspired-ai
WORLD SUMMIT AI @ INSPIRED AI
14 October 2020
Online
WORLD AI WEEK
12-16 October 2020
Amsterdam, Netherlands
INTELLIGENT HEALTH UK
3-4 March 2021
London, UK
WORLD SUMMIT AI AMERICAS
20-21 April 2021
Montreal, Canada